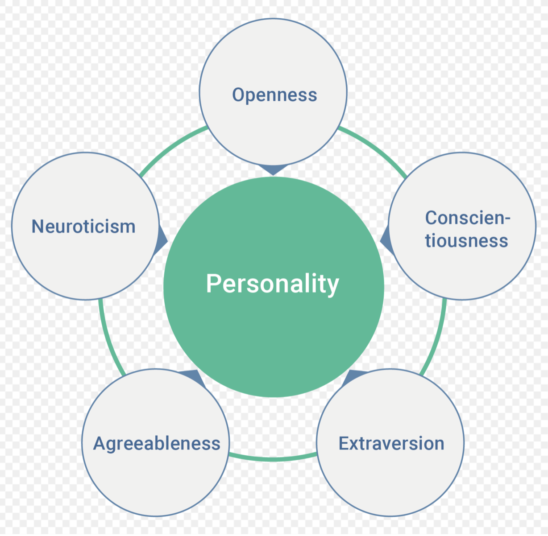
This is the second post reviewing articles in the journal Distance Education, Vol. 40, No.3, a special edition on learning analytics in distance education. The first article was on analytics and learning design at the UKOU. As always if you find these posts of interest, please read the original articles. Your conclusions will almost certainly be different from mine.
Wu, F. and Lai, S. (2019) Linking prediction with personality traits: a learning analytic approach Distance Education, Vol. 40, No.3
Aim of research
This study examines using two of the Big Five personality traits (Costa and Macrae, 1992):
- extraversion
- openness to experience
to predict student achievement in blended learning in Chinese middle schools, using the statistical technique of multiple regression, and selected Education Data Mining algorithms. The aim was to identify at-risk students so timely interventions can in future be made to prevent learning failure.
The authors state that the Big Five personality traits reflect ‘learners’ unparalleled and relatively stable patterns of behaviour, thoughts and emotions’ (even in teenagers).
Methodology
The study involved 662 students taking a blended mathematics course over a 19 week semester in a Chinese senior high school.
Student achievement was measured by student activity in the LMS, identified in four categories:
- attendance (frequency online)
- use of resources (time spent on LMS/videos)
- participation in discussion forum
- assignment scores
Student achievement measurements were made at four points during the semester: 5th, 9th, 15th and 19th week
Student personality traits (extraversion/openness) were identified through a Big Five questionnaire (NEO-FFI). Students were allocated to four groups, depending on their combination of scores on the two personality traits selected.
Results
Achievement prediction accuracy gradually increased for each of the ‘five groups during the four chosen weeks’. The performance of the deep learning algorithms (DBN in particular) was better than multiple regression alone or other baseline algorithms in prediction accuracy.
‘The present study is pioneering in…showing that personality traits need to be considered when using learning behaviours as predictors for achievement’. ‘Prediction accuracies when grouping students were more accurate than when treating them as a whole group.’
Comment
Where do I start? I find this a really scary study. First, as the article itself notes, no interventions were planned so the effectiveness of intervening to help students at risk is not even examined.
Also, what does ‘at risk’ mean? Would this be students generally with the ‘wrong’ personality traits (or combination) or is it individual students within each personality trait being identified (I hope it is the latter)? Some groups were more accurately predictable than others, but was there any difference in the actual group achievements? We’re not told – this was not of interest to the study. But what if those with an extravert personality were found to be more at risk? What are the consequences of this finding, especially in a country such as China?
On the other hand, I suspect that all this study tells us is that half-way through a course, some students are doing worse than others, and these are more likely to fail at the end. But couldn’t that be determined by looking at their actual assessment scores at a midpoint in the course?
Thirdly prediction becomes gradually more accurate as the semester progresses, but by the time accuracy gets to 75% (the 15th week for three of the four personality clusters) it is probably too late to undertake a major ‘intervention’. And what about the 25% or so (165 students) who are not accurately predicted? What does one do for them? What if an intervention was made and they didn’t need it?
Also what do the algorithms actually do? The ‘deep learning’ DBN algorithm was the most accurate predictor. Why? Is there something about the algorithm that can tell us what needs to be done to help students or their teacher? As with most AI/learning analytics papers, the way the algorithms function is never explained (and even if it was, it would probably make no sense to an ‘intervener, i.e. the teacher or student.) There must be some way though to relate the algebra in an algorithm to external, real world explanations.
Perhaps the most disturbing thing about this kind of study (and it is by no means isolated) is the underlying cultural assumptions about learning and teaching. Failure is an essential part of learning. We learn by our mistakes. It is the role of the teacher to identify and help students who would otherwise fail. Being ‘at risk’ is not an evil to be fixed but a source of hope. Identifying students at risk without relating the ‘risk’ to successful pedagogical interventions is unethical (talk to the medical profession about this). Students are individuals and as such need to be treated individually. Education is not a filtering exercise. This was not the intention of the study but the technology and the thinking behind it lends itself to that type of application.
Lastly, I may well have failed to understand properly this paper. But this raises a real question. If there is something practical for teachers/instructors or students in this paper, I failed to identify it. If there is something significant in this study, we are getting to the point where educational experiments are being conducted in such a way that it is not possible to explain to teachers or students what is actually going on or what to do about it. Just run the algorithms and see what results. And that is the scariest part for me.
Sleep well, sweet innocent souls.
Next up
Use of learning analytics to identify social network patterns in game-based learning
Reference
Costa, P. and Macrae, R. (1992) Revised NEO personality inventory (NEO-PI-R) and NFO Five-Factor Inventory (NEO-FFI): Professional Manual Odessa FL: Psychological Assessment Resources