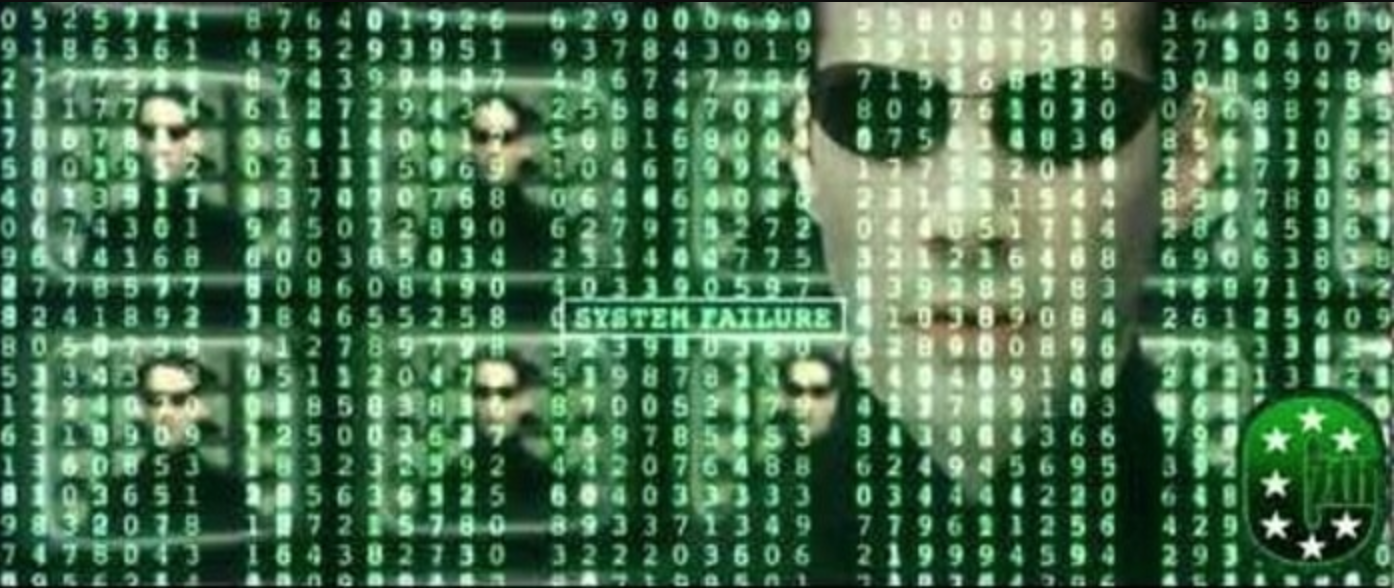
I have now covered all five main articles in the special August issue of the journal Distance Education on learning analytics (because it is not an open publication). These are:
- analytics and learning design at the UKOU.
- analytics and personality traits in a high school in China
- analytics and gamification in an undergraduate course at a Hong Kong university
- learning analytics to predict mastery using an online mathematics tutoring system
- MOOCs and student data privacy: a sentiment analysis based on the terms of service of three MOOC providers.
However, there are also two commentaries on the five articles in this issue, one by George Siemens and the other by Michael Jacobson. I will briefly discuss these commentaries then give my own overview of what the five papers suggest to me about the current use of learning analytics in online learning and distance education.
George Siemens’ commentary
I will deal with this briefly, as George mainly explains clearly the purpose or potential of each of the studies, and the importance of the questions they raise for the future, but he does not address the actual results of each study.
I agree with George that learning analytics have great potential, but the issue for me is what are learning analytics actually delivering at the moment within the five reported studies and this was not really addressed in George’s commentary.
Michael Jacobson’s commentary
Michael Jacobson noted the diverse range of topics covered by the five research studies (a point I will comment on further below), even though all the studies are within the area of open, flexible and distance learning. He looks for what ties such diverse topics together. In doing so, he touches on what I consider to be the essential issue in many if not all studies using learning analytics. As Jacobson puts it:
LA methods that primarily identify ..patterns in data….are distinct from theories that propose explanatory perspectives for how and why identified patterns of behaviour might have occurred as well as probabilistic likelihoods such patterns and behaviors might occur in the future.
He then goes on to propose a theoretical framework for designing and interpreting learning analytics studies (Jacobson et al, 2019). In particular, he emphasises a particular view of learning that is often missing in LA and more general AI studies. Basically Jacobson argues that LA studies too often focus on micro levels of learning through simple multiple-choice questions, or (even worse) measurement of behaviours that act as substitutes for the measurement of learning, such as number of interactions with an LMS, and ignore the more macro levels of learning that include cognitive processing, such as building explanatory schema and mental models. In particular he sees learning as ’emergent’ (and what I call developmental).
Jacobson describes three types of learning identified by the (U.S.) National Research Research Council (2001):
- declarative knowledge: factual (e,g. names of flowers, parts of the body)
- procedural knowledge: knowing how to do a task
- explanatory knowledge: being able to explain why.
Indeed, he goes even further to argue that open, flexible and distance learning systems have difficulties measuring the latter two forms of learning using quantitative machine-scorable assessment.
He then goes on to explain his complex systems conceptual framework of learning (CSCFL) which takes account of the complexity of educational systems and student learning. In essence he is arguing that without theory, LA researchers can’t see the forest for the trees.
There is more detail in Jacobson’s paper, but I totally agree with his view that LA is unlikely to deliver useful results if the researchers do not have a clear understanding of the nature of learning, and that data without theory or explanation are not going to improve student learning. Anyone considering doing learning analytics should read Jacobson’s article first.
Main lessons from this edition
First, I would like to congratulate the editors (Jingjing Zhang, Daniel Burgos, and Shane Dawson) for putting together this edition. It does provide more than a glimpse of the kind of studies being carried out using learning analytics in the field of online, flexible and distance learning, although I should point out that there is a journal, The Journal of Learning Analytics devoted to more general LA applications. This has the advantage of being an open access journal and has many more cases of LA applications than the Distance Education edition. I am not though in a position to know how typical the five studies in Distance Education are, although they reflect similar approaches in a range of studies of AI in higher education that I have encountered.
So what do I take away from this edition? I recognise that five studies is a very small sample so these comments refer only to the articles accepted for this edition.
1. Disappointing results
Despite the hype and the promise, only one of the five studies came up with a significant result that provides guidelines on teaching and learning resulting directly from the application of learning analytics. This was the study by Huang et al. on gamification. Interestingly this was a classic control-group experiment that found gamification resulted in greater communication between students, better quality online postings and better learning outcomes. It is interesting that this was the only one of the five studies that proposed an approach for the intervention based on a learning theory. However, I found it difficult to see how the method of this study differed from a traditional statistical analysis of a quasi-experimental design, with the important exception of the use of LA to identify patterns in students’ communication, where LA proved valuable.
There was an interesting result from the Holmes et al paper on learning design at the UK Open University. They found that most of the modules/courses following the OU’s learning design process were heavy on instructional (instructor-directed) activities such as assimilation (reading, or viewing videos) and assessment, and were relatively light on the more learner-centred activities such as production and experience. That is a useful result in its own right, but the study found no statistical differences in student outcomes between the 55 different applications of learning design at the OU, probably because there wasn’t a very meaningful variation in designs. This approach though definitely warrants another try where there are more variations in learning design.
The Wu and Lai paper on using personality traits to predict student success in high school mathematics in China was able to increase the accuracy of prediction as a course progressed but it was not until three quarters of the way through the course before the predictions reached an accuracy of 75%, and it was not clear from the study whether certain personality traits were more likely to lead to success than others. The main result was identifying which of two algorithms was the more accurate: great for further LA studies but not very helpful for improving student learning.
The Slater and Baker paper that attempted to forecast mastery of learning from the number of learners’ attempts at math problems found one algorithmic prediction method useless but found another (PFA) able to predict the number of attempts needed to get the answer correct within two or three attempts (out of a maximum of 15). I have been trying to think ever since I read it what the value of this is.
Lastly, Prinsloo et al. asked the very interesting question as to whether the terms of service of MOOC providers are laden with emotive language that will mislead students into giving away their personal data. Unfortunately though the sentiment analysis found the MOOC providers not guilty, or at least the evidence wasn’t there. The study also indicated that a traditional critical content analysis of the service agreements would have been better than the use of learning analytics based on automated lexicons.
Thus, as with many research studies, the most interesting findings were not the ones the researchers were initially looking for. In terms of evidence of the power of learning analytics, though, the five papers were unimpressive.
2. The need for theory or hypotheses
This is probably the main issue in the application of AI and/or learning analytics in education. Merely identifying statistical patterns of behaviour is not enough in itself to lead to better student learning. There is a gap between identifying patterns and the actions needed to identify or address the issues resulting from the identification of the pattern. However, this is the goal (or dream) of AI: that the patterns themselves are self-explanatory. They are not. One of three questions needs to be answered:
- what should a student do as a result of the analysis?
- what should a teacher/instructor do as a result of the analysis?
- what should an administrator do as a result of the analysis?
To answer any of these questions you need to have a theory of learning: how do students learn? Measuring what students do (such as getting a multiple-choice question right or wrong or clicking on a web page) is not the same as measuring their learning. Measuring learning is difficult for reasons laid out in the Jacobson commentary. Correlation is not the same as cause. Too often this is the mistake made in learning analytics. Find a correlation and we have an explanation. And too often the main aim of LA studies is not to answer the questions above but to find the best ‘tool’ for ‘prediction’.
3. The gap between LA and the reality of teaching and learning
When I read these papers, they seem so far removed from the reality of teaching and learning, either in a classroom or online. They are like machines running in their own universe that has nothing to do with the realities of teaching and learning. They are in most cases measuring the wrong things, mainly things that are both easy to count and are frequently occurring, but do not reflect phenomena that represent the actual learning or teaching process. Above all they do not respect the importance of human connections and agency.
4. The importance of respecting values in education
In at least two of the papers the authors misunderstand what I believe to be the purpose of education. The aim is not to be efficient and weed out the weak learners from the system, but to provide every learner with the best education possible under the circumstances. Providing an analysis of learning difficulties without relating the analysis to means of overcoming the difficulties is amoral. This is where the analysis of data needs to be informed by the principles and values of education. Don’t identify a student with a learning ‘deficit’ unless you have at least a possible solution that is more than ‘Go back and do it again.’
5. Trying hard but needs to do better
Don’t get me wrong. I think there is a value in analysing the massive amounts of data that are digitally generated in online teaching and learning. But the analysis must be driven by valid measurements of learning, by learning theory and hypotheses based on prior knowledge of how learning develops, and most of all by a concern for the values of education.
I would also apply the criterion of return on investment to these studies. They seem to involve an inordinate amount of work to collect and analyse the data and so far the results are meagre, to say the least. In at least two cases, traditional research methods would have provided better results.
I also have the feeling that sometimes there are useful results, but the analytical procedures are so obtuse and complicated that they cannot be easily explained. However, as a teacher, I need to know why I need to change and as a student I need to know (and more importantly should have the right to know) why I am denied access to a course.
Thus it is important to choose the right kinds of projects for learning analytics rather than measuring and analysing any kind of available data, just because it’s there. Above all, computer scientists need to work with educators if learning analytics are ever to prove useful in practice.
References
Jacobson, M. et al. (2019) Education as a complex system: conceptual and methodological implications Educational Researcher, Vol. 48, No. 2
National Research Council (2001) Knowing what students know: the science and design of educational assessment Washington DC: The National Academies Press
Hi Tony,
Thanks for the commentary. The field of Learning Analytics is navigating the usual Gartner hype cycle curve, in which the rigorously thought through examples are naturally eclipsed by the more prevalent poorer examples that come from products that count what can be easily measured, but don’t measure what really counts in learning and teaching. C’est la vie, and only to be expected in a field with high injections of tech $$.
FYI…Questions of how educational/learning sciences theory inform analytics, how such tools are integrated into pedagogical practice, how stakeholders can be given a real voice in the design process, and how design teams tackle political/ethical concerns, are being tackled head-on by the community. Naturally, this more careful approach is slower out of the blocks than those build first and ask questions later, so the imperative has never been greater for informed critique to move from shouting from the sidelines, to *shape design* in practical ways. We’re working on it 🙂
e.g.
On human-centred design: https://twitter.com/sbuckshum/status/1124608589577039872
On the importance of theory: https://epress.lib.uts.edu.au/journals/index.php/JLA/issue/view/358
On atheoretical dashboards: https://doi.org/10.1145/3170358.3170421
On aligning analytics with learning design, and its impact: https://antonetteshibani.com/wp-content/uploads/2019/01/Shibani-et-al-2019-Contextualizable-Learning-Analytics-Design_authors-copy.pdf
Simon
Thanks for another thought-provoking post Tony!
Also thanks to Simon for his comment. I sense there is an actual shift and interest towards this human-centered (or user/student-centered) approach of understanding LA. This also comes forth in recent and not-so recent papers such as
Broughan, C., & Prinsloo, P. (2019). (Re)centring students in learning analytics: in conversation with Paulo Freire. Assessment & Evaluation in Higher Education, 1–12. https://doi.org/10.1080/02602938.2019.1679716
Tsai, Y., Perrotta, C., & Gašević, D. (2019). Empowering learners with personalised learning approaches? Agency, equity and transparency in the context of learning analytics. Assessment & Evaluation in Higher Education. https://doi.org/10.1080/02602938.2019.1676396
Slade, S., & Prinsloo, P. (2013). Learning Analytics: Ethical Issues and Dilemmas. American Behavioral Scientist, 57(10), 1510–1529. https://doi.org/10.1177/0002764213479366
West, D., Searle, B., Vanderlelie, J., Toohey, D., Luzeckyj, A., Bell, K., … Toohey, D. (2019). Learner Facing Analytics Analysis of Student Perspectives. Melbourne.
Still, I am not entirely sure if we really understand “human-centered” the same or even similar way, and how deeply we actually think about it or allow our research approaches to lead. If for example we look at Tsai et al. 2019 article, its methodology of focus groups and thematic analysis are sort of doing it. Our research project did the same thing in Finland with 11 HEIs and close to 150 students also this year.
After reading Broughan & Prinsloo (2019) article, among other things, I actually started to question how “human” or student-centered our focus group research really was? Could it have gone even deeper and wider to really understand the students’ experience and the various relations between them and data and technologies? This is what for example various forms of critical research “methods” such as critical ethnography, socio-analysis and others could achieve, but often we don’t have enough time, skills or even consider them as “real” research. I feel that output from such studies would be especially important because what we are inherently dealing with here are e.g. various kinds of power-relations, what the designers of LA systems consider to be important (in relation to learning, but other matters too such as participation and ownership) and several other “invisible” matters that go way beyond “did they click that or not?” and “based on the prediction should we help/kick these people out?” or worse, before even accepting them in, “should we keep them out as their prediction looks really bad?”.
I think Broughan & Prinsloo (2019) put it well, that there is a danger that we develop based on prejudice that are hidden from ourselves (for example as white Western people with certain worldviews). And that is why I believe it is important for the “LA community” to be open to various views and ways of seeing, and studying phenomena around LA and data. It is those people who are uninterested about these topics but possibly affected by our doings that we need to hear and “see”, together with them.
But as I wrote, I sense there is a shift towards deeper dialogue in these topics, so I am hopeful. Sorry for the long post, yeah I’ve heard no one reads anymore, but this is writing as well it is thinking at the same time.
Many thanks, Marko, for a great comment. No need to apologise for thinking out loud.
There does seem a willingness to think about the humanistic issues in the application of learning analytics and to a lesser extent in AI applications. But as you say, the power dimension here are important. Who decides (a) what to analyse (b) how the data should be analysed (c) what to do with the results? The worry I have is that the individual (learner or student) is the weakest in this chain of power, and the teacher or instructor is the next weakest. It worries me that it is often computer scientists without an understanding of what learning is and how it is acquired who are making the decisions about (a) and (b) and it is administrators and even politicians who are doing (c). How do we get learners and educators to play a more influential role in the applications of learning analytics?